Designing an AI-driven shopping app reducing overwhelm and driving confident purchases
Designing an AI-driven shopping app reducing overwhelm and driving confident purchases
Designing an AI-driven shopping app reducing overwhelm and driving confident purchases



Context
FOMO is an early stage start-up founding an AI-powered fashion marketplace set to launch in June - July 2025. When I came in, the co-founders had strong market insights and dozens of exciting features on the roadmap. They needed my help to bring clarity and usability to a very ambitious idea.
This case study explores how I transformed complexity into an AI-driven shopping app that reduces overwhelm—streamlining onboarding, guiding discovery, and enabling confident purchases
This case study explores how I transformed complexity into an AI-driven shopping app that reduces overwhelm—streamlining onboarding, guiding discovery, and enabling confident purchases
This case study explores how I transformed complexity into an AI-driven shopping app that reduces overwhelm—streamlining onboarding, guiding discovery, and enabling confident purchases
[Type]
E-Commerce
[My Role]
Lead Designer
[Timeline]
January 2024- March 2024
[Team]
Founder, PM, Engineering Team
[Team]
Cofounders, Engineering Team
✨ Desktop recommended
Heads-up: images are smaller and layout seems longer on mobile—view on desktop for best clarity!
Your interest means everything—thanks for exploring my work! 🌟"
The Problem
Most shopping apps flood users with endless options—causing frustration, decision fatigue, and drop-off. FOMO was at risk of doing the same, despite having smarter tech.
The Outcome
I designed the shopping app with a focus on human-centered AI—using lean UX to simplify decision-making, improve onboarding, and build trust in recommendations for a faster, clearer, and more enjoyable shopping experience.
The Impact
✅ SUS score of 83.5 (excellent usability)
✅ 2× faster product discovery
✅ 60% of users said AI recommendations increased trust
✅ Helped secure pre-seed funding

Navigation: High-Fidelity Prototype
The Impact
✅ SUS score of 83.5 (excellent usability)
✅ 2× faster product discovery
✅ 60% of users said AI recommendations increased trust
✅ Helped secure pre-seed funding


Navigation: High-Fidelity Prototype
The Impact
✅ SUS score of 83.5 (excellent usability)
✅ 2× faster product discovery
✅ 60% of users said AI recommendations increased trust
✅ Helped secure pre-seed funding
Here’s how I brought focus to a scattered vision
Before we could build user trust, we had to bring clarity to the product.
I started by translating the founders’ vision into a low-fidelity flow—featuring outfit planners, community boards, and social voting. While ambitious, the experience was overloaded. Once visualized, it became clear: we weren’t building a helpful assistant; we were overwhelming users with complexity.
This quick mockup became a turning point. It helped the team see that starting with everything meant delivering nothing well.
This quick mockup became a turning point. It helped the team see that starting with everything meant delivering nothing well.


Fig. Visualizing Founder's Vision into a Low-Fid Mockup
My Pivot Pitch
I proposed a reset grounded in user needs:
✅ Mapped real user pain points—showed founders how extra features would worsen decision fatigue.
✅ Proposed a phased approach—start with core issues (personalization + outfit visualization), prove value, then scale.
✅ Co-created a lean roadmap—simplify MVP, measure engagement, then add "delight" features.
To validate this direction, I conducted discovery research with target audience (ages 18–35) and mapped the emotional arc of their shopping journey—from discovery to purchase.
To validate this direction, I conducted discovery research with target audience (ages 18–35) and mapped the emotional arc of their shopping journey—from discovery to purchase.
JOURNEY STAGES:
1
Discovery
2
Browse / Search
3
Save / Shortlist
4
Purchase
1
Discovery
2
Browse / Search
3
Save / Shortlist
4
Purchase
Excited
Excited
Doubtful
Doubtful
Hopeful
Hopeful
Frustrated
Frustrated
Abandon
Abandon
USER PAINS:
"I save tons of inspiration posts, but can’t find anything similar in my budget on shopping apps."
"I save tons of inspiration posts, but can’t find anything similar in my budget on shopping apps."


Anita (19, Student)
"Even after applying filters, I scroll endlessly through irrelevant options."
"Even after applying filters, I scroll endlessly through irrelevant options."


Alexis (26, Professional)
"I keep second-guessing my wishlist—I’m stuck in analysis paralysis."
"I keep second-guessing my wishlist—I’m stuck in analysis paralysis."
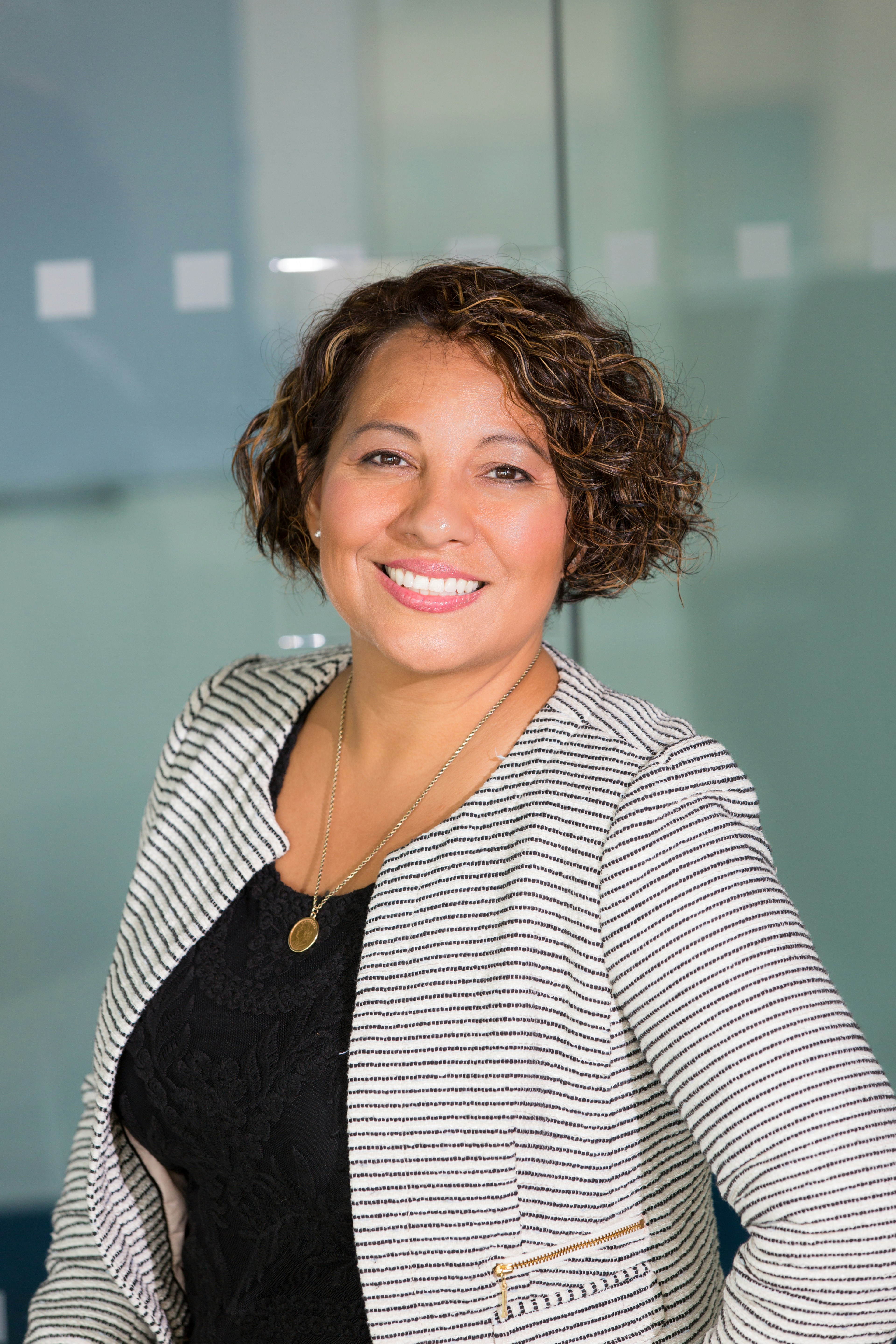
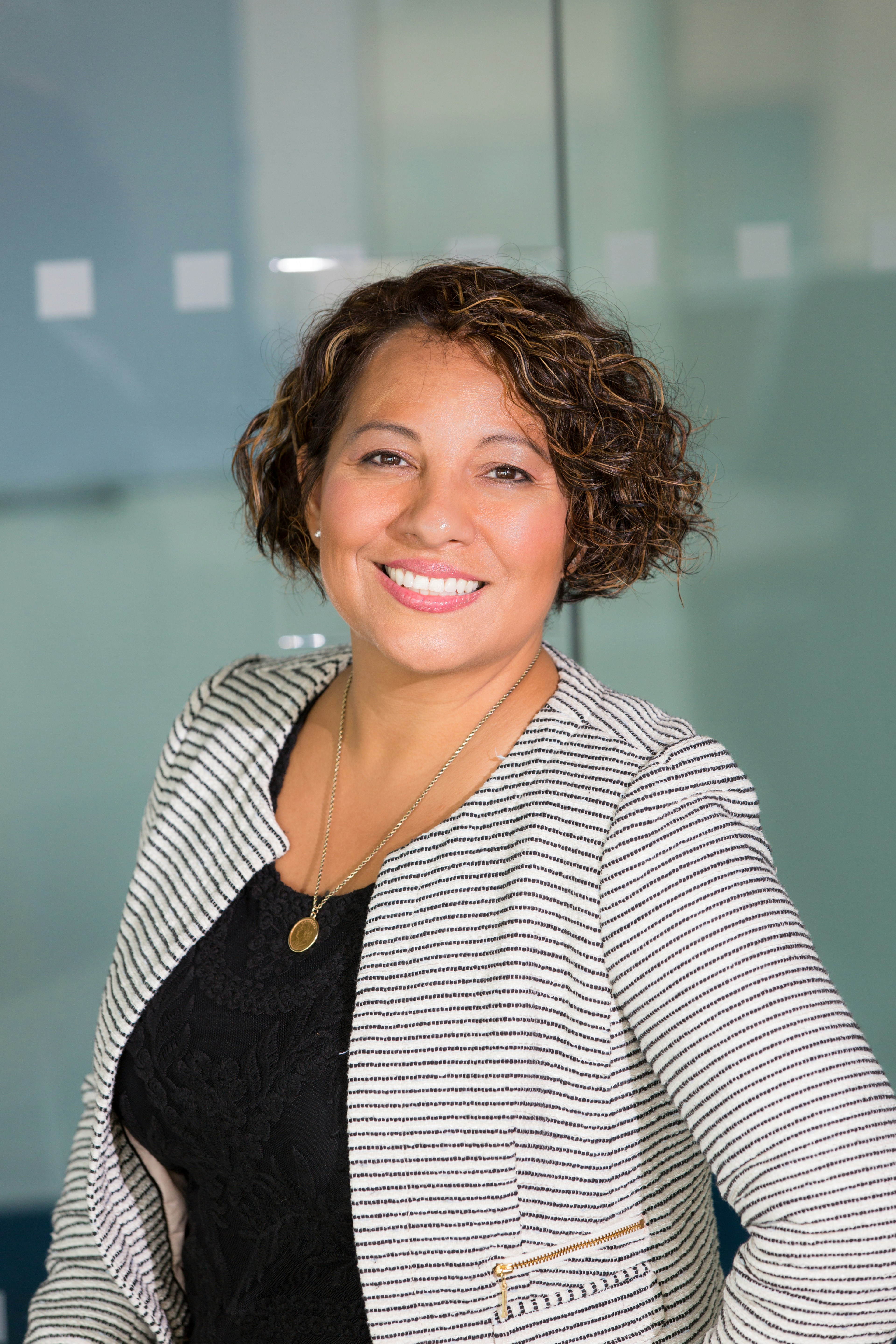
Jenny (32, Business Owner)
Designing for Confidence
Once we had clarity on what not to build, the real work began—designing a product that could guide users without overwhelming them.
Once we had clarity on what not to build, the real work began—designing a product that could guide users without overwhelming them.
I framed the roadmap around one simple idea: give users just enough help to feel confident, without taking away their sense of control.
I framed the roadmap around one simple idea: give users just enough help to feel confident, without taking away their sense of control.
Login /
Signup
Onboarding
questionnaire
Model learns
user preferences
Curated items
displayed
Decision
making help
Checkout
Flow 1 - Onboarding that feels like a stylist, not a survey
To make the AI recommendations meaningful, we needed high-quality inputs—preferences around body type, skin tone, and style. But asking those questions too soon—or the wrong way—could trigger drop-off or discomfort.
The tone was friendly. The visuals were inclusive. And the questions—about body type, skin tone, and style—were explained clearly so no one felt confused.
The tone was friendly. The visuals were inclusive. And the questions—about body type, skin tone, and style—were explained clearly so no one felt confused.
Most importantly, it was optional.
If users didn’t feel comfortable sharing upfront, they could skip and personalize on their terms. The entire app’s personalization relied on this moment—so building trust from the first tap was everything.


Fig. Screens from Onboarding
Designing for Confidence
Flow 2 - A curated feed built on restraint
The homepage / search results page was designed to focus.
Inspired by cognitive science (like Miller’s Law), I limited the feed to just four items at a time—each one tailored to the user’s preferences. No infinite scroll. No clutter.
Inspired by cognitive science (like Miller’s Law), I limited the feed to just four items at a time—each one tailored to the user’s preferences. No infinite scroll. No clutter.
Even small details mattered: if an item was out of stock or the wrong size, we simply didn’t show it—unless the user asked. The goal wasn’t to show everything. It was to show what mattered.
With each swipe—save, skip, add to cart—the AI got smarter. But more importantly, users felt more in control.


Fig. Screens for Search & Browse
Flow 3 - Building trust into AI in every choice
This was where most shoppers got stuck—at the decision point.
To ease that moment, I designed a swipeable outfit preview carousel. Users could see how saved tops and bottoms looked together before committing. No guesswork.
To ease that moment, I designed a swipeable outfit preview carousel. Users could see how saved tops and bottoms looked together before committing. No guesswork.
Each recommendation came with a reason—"Based on your style and recent likes..."—to make the AI feel less random and more like a smart assistant.
And when automation got it wrong? Manual overrides were always one tap away. Because good AI doesn’t just predict—it listens.


Fig. Screens for Wishlist and AI Trust
Designing for trust wasn’t just a principle—it had to be proven
After shaping the core flows, I tested each touchpoint with real users to ensure it felt intuitive, helpful, and worth coming back to.
After shaping the core flows, I tested each touchpoint with real users to ensure it felt intuitive, helpful, and worth coming back to.
Could a few well-asked questions change how people shop?
Could a few well-asked questions change how people shop?
"I enjoyed answering these questions - it was so hard finding outfits for my body type before. Now I know I won't waste time on irrelevant clutter later."
"I enjoyed answering these questions - it was so hard finding outfits for my body type before. Now I know I won't waste time on irrelevant clutter later."


Anita (19, Student)
Impact:
✅ 2.5× more engagement with image-driven style selections
✅ 2.5× more engagement with image-driven style selections
✅ 1 in 3 skippers returned to complete onboarding later
✅ 1 in 3 skippers returned to complete onboarding later


High-Fidelity Prototype - Onboarding


High-Fidelity Prototype - Browsing
What happens when you stop showing users everything?
What happens when you stop showing users everything?
“This is what I’d normally spend hours saving. But it’s all here—already.”
“This is what I’d normally spend hours saving. But it’s all here—already.”


Alexis (26, Professional)
Impact:
✅ Users found products 2× faster than traditional browsing
✅ Users found products 2× faster than traditional browsing
✅ 60% said AI reasoning helped them avoid impulse purchases
✅ 60% said AI reasoning helped them avoid impulse purchases
The hardest part? Deciding. Did it become easier?
The hardest part? Deciding. Did it become easier?
“The outfit builder fixes everything I struggle with—seeing how it all works together.”
“The outfit builder fixes everything I struggle with—seeing how it all works together.”
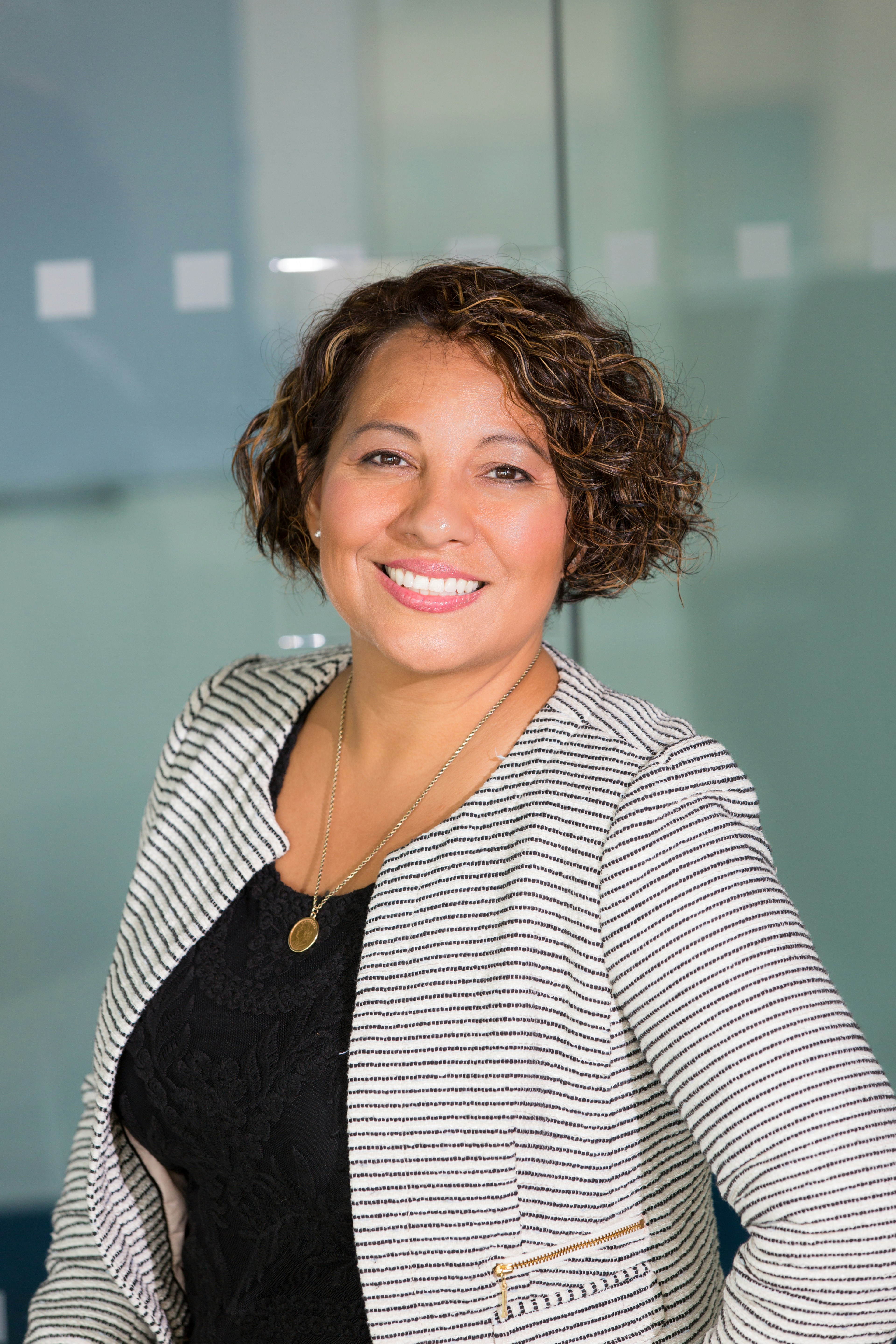
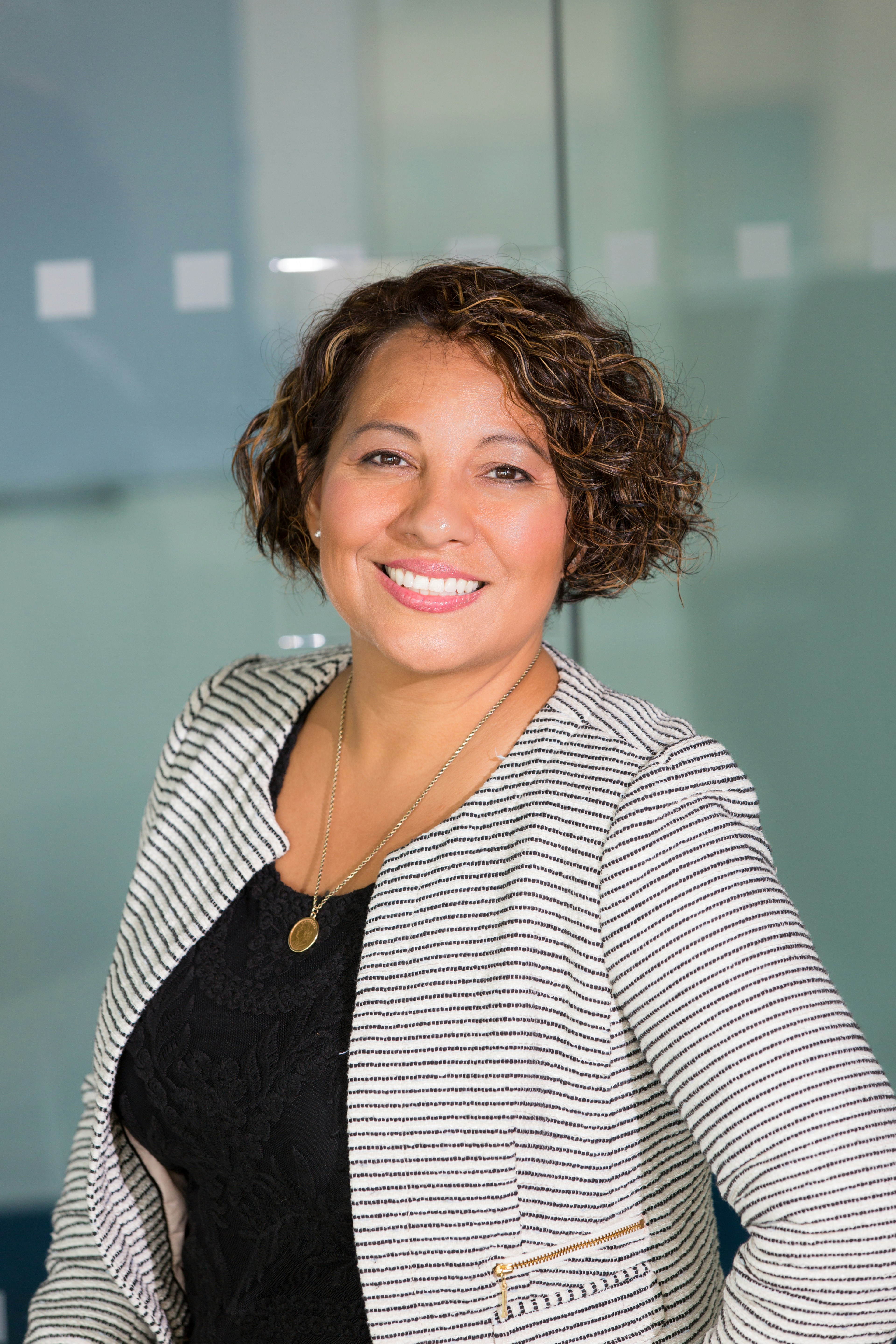
Jenny (32, Business Owner)
Impact:
✅ Users were more likely to purchase full looks when they could preview outfits
✅ Users were more likely to purchase full looks when they could preview outfits
✅ SUS score: 83.5 — excellent usability
✅ SUS score: 83.5 — excellent usability


High-Fidelity Prototype - Shortlisting + Purchase
Finally, I shipped a cohesive MVP that scales with user needs
I balanced speed and structure—designing 200+ screens and micro-interactions with an emphasis on rapid validation and ethical AI considerations.
I maintained a clean, vibrant visual language—soft pastels with black accents—to reduce visual noise and draw focus to product imagery.
I maintained a clean, vibrant visual language—soft pastels with black accents—to reduce visual noise and draw focus to product imagery.
I used token-based components and an adapted design system to accelerate iteration while ensuring consistency
Cross-functional alignment:
✅ Weekly syncs with PM, engineers, and the founder kept design aligned with tech constraints and product vision
✅ Detailed annotations for complex interactions (e.g., "swipe-to-train") to bridge design-dev gaps
✅ Built modular foundations—scalable patterns that could evolve beyond the MVP without redesign
Once we had clarity on what not to build, the real work began—designing a product that could guide users without overwhelming them.
I framed the roadmap around one simple idea: give users just enough help to feel confident, without taking away their sense of control.
Login /
Signup
Onboarding
questionnaire
Model learns
user preferences
Curated items
displayed
Decision
making help
Checkout
Flow 1 - Onboarding that feels like a stylist, not a survey
To make the AI recommendations meaningful, we needed high-quality inputs—preferences around body type, skin tone, and style. But asking those questions too soon—or the wrong way—could trigger drop-off or discomfort.
The tone was friendly. The visuals were inclusive. And the questions—about body type, skin tone, and style—were explained clearly so no one felt confused.
Most importantly, it was optional.
If users didn’t feel comfortable sharing upfront, they could skip and personalize on their terms. The entire app’s personalization relied on this moment—so building trust from the first tap was everything.


Fig. Screens from Onboarding
Flow 2 - A curated feed built on restraint
The homepage / search results page was designed to focus.
Inspired by cognitive science (like Miller’s Law), I limited the feed to just four items at a time—each one tailored to the user’s preferences. No infinite scroll. No clutter.
Even small details mattered: if an item was out of stock or the wrong size, we simply didn’t show it—unless the user asked. The goal wasn’t to show everything. It was to show what mattered.
With each swipe—save, skip, add to cart—the AI got smarter. But more importantly, users felt more in control.


Fig. Screens for Search & Browse
Flow 1 - Onboarding that feels like a stylist, not a survey
To make the AI recommendations meaningful, we needed high-quality inputs—preferences around body type, skin tone, and style. But asking those questions too soon—or the wrong way—could trigger drop-off or discomfort.
The tone was friendly. The visuals were inclusive. And the questions—about body type, skin tone, and style—were explained clearly so no one felt confused.
Most importantly, it was optional.
If users didn’t feel comfortable sharing upfront, they could skip and personalize on their terms. The entire app’s personalization relied on this moment—so building trust from the first tap was everything.


Fig. Screens from Onboarding
Designing for trust wasn’t just a principle—it had to be proven
After shaping the core flows, I tested each touchpoint with real users to ensure it felt intuitive, helpful, and worth coming back to.
Could a few well-asked questions change how people shop?
"I enjoyed answering these questions - it was so hard finding outfits for my body type before. Now I know I won't waste time on irrelevant clutter later."

Anita (19, Student)
Impact:
✅ 2.5× more engagement with image-driven style selections
✅ 1 in 3 skippers returned to complete onboarding later

High-Fidelity Prototype - Onboarding
What happens when you stop showing users everything?
“This is what I’d normally spend hours saving. But it’s all here—already.”

Alexis (26, Professional)
Impact:
✅ Users found products 2× faster than traditional browsing
✅ 60% said AI reasoning helped them avoid impulse purchases

High-Fidelity Prototype - Browsing
The hardest part? Deciding. Did it become easier?
“The outfit builder fixes everything I struggle with—seeing how it all works together.”
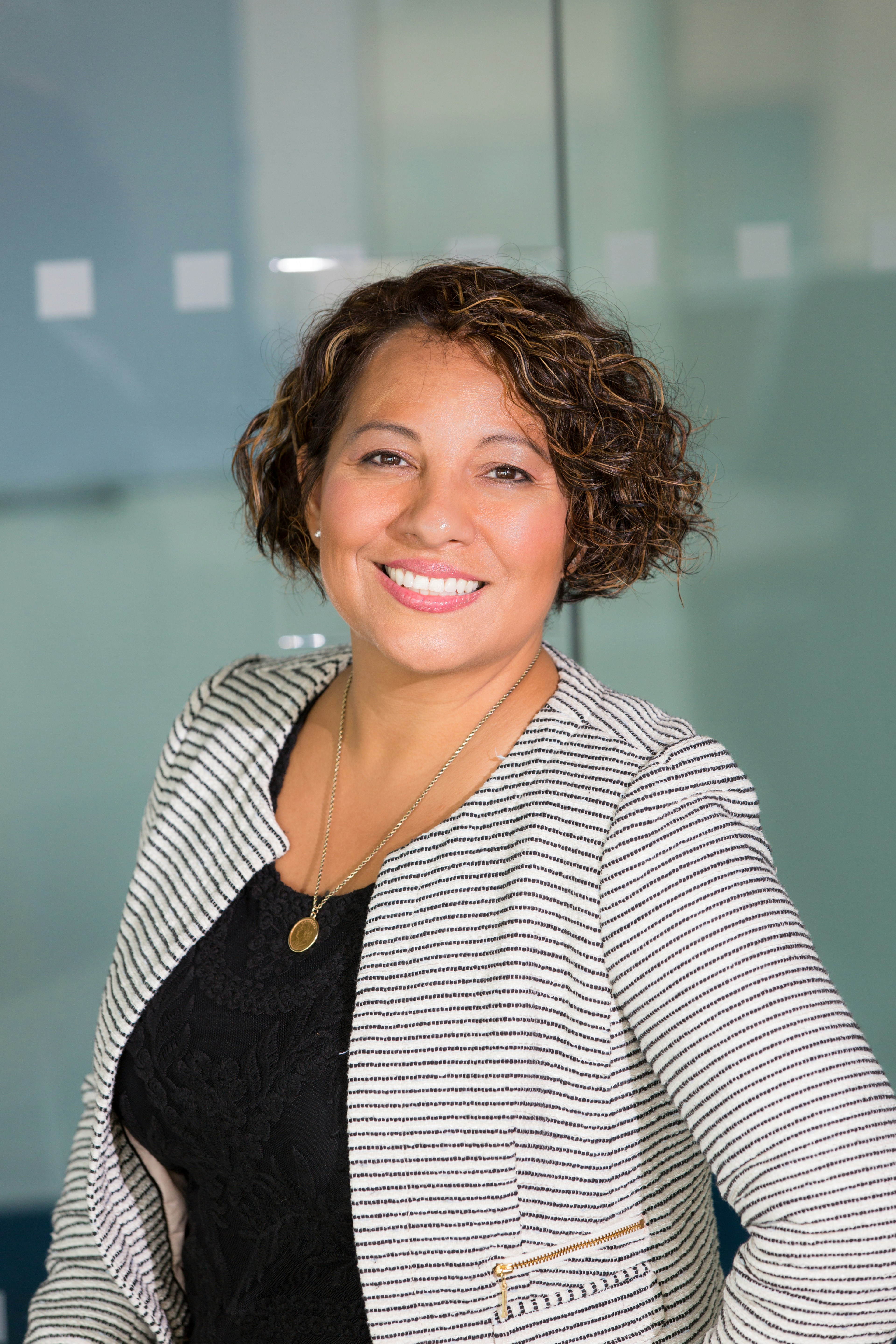
Jenny (32, Business Owner)
Impact:
✅ Users were more likely to purchase full looks when they could preview outfits
✅ SUS score: 83.5 — excellent usability

High-Fidelity Prototype - Shortlisting + Purchase
Finally, I shipped a cohesive MVP that scales with user needs
I balanced speed and structure—designing 200+ screens and micro-interactions with an emphasis on rapid validation and ethical AI considerations.
I maintained a clean, vibrant visual language—soft pastels with black accents—to reduce visual noise and draw focus to product imagery.
I used token-based components and an adapted design system to accelerate iteration while ensuring consistency
Cross-functional alignment:
✅ Weekly syncs with PM, engineers, and the founder kept design aligned with tech constraints and product vision
✅ Detailed annotations for complex interactions (e.g., "swipe-to-train") to bridge design-dev gaps
✅ Built modular foundations—scalable patterns that could evolve beyond the MVP without redesign
Key Learnings
Designing AI with transparency builds trust
Designing AI with transparency builds trust
I learned that users don’t just want smart suggestions—they want to understand them. By surfacing the “why” behind AI recommendations, I made the system feel collaborative rather than opaque, helping users feel more in control of their decisions.
MVP ≠ Minimal Design — it’s Focused Design
MVP ≠ Minimal Design — it’s Focused Design
Shipping 200+ screens while staying true to MVP scope taught me how to make smart tradeoffs—prioritizing core flows, documenting edge cases, and building modular designs that could evolve without starting over.
Human-centered AI means designing for decision support—not automation
Human-centered AI means designing for decision support—not automation
Rather than aiming to replace user effort, I focused on supporting key decision points—through curated options, visual outfit builders, and override flexibility. Good AI design empowers, not overwhelms.
Thank you for reading.

[Next Project]
Designing a fraud detection dashboard to boost manager oversight and analyst productivity
Data Visualisation
Gamification
Interaction Design
View Full Case Study
Key Learnings
Designing AI with transparency builds trust
I learned that users don’t just want smart suggestions—they want to understand them. By surfacing the “why” behind AI recommendations, I made the system feel collaborative rather than opaque, helping users feel more in control of their decisions.
MVP ≠ Minimal Design — it’s Focused Design
Shipping 200+ screens while staying true to MVP scope taught me how to make smart tradeoffs—prioritizing core flows, documenting edge cases, and building modular designs that could evolve without starting over.
Human-centered AI means designing for decision support—not automation
Rather than aiming to replace user effort, I focused on supporting key decision points—through curated options, visual outfit builders, and override flexibility. Good AI design empowers, not overwhelms.

Anita (19)
Student in Big City
"I save so many inspiration posts that match my style on Insta and Pinterest but I rarely find the exact ones in my budget on my usual shopping apps.”
Saves 15+ inspo posts on social media weekly, but only converts 2-3 to purchases / month.

Alexis (26)
Working Professional
"I spend hours searching for trendy outfits—filtering by size, my style, reviews. But even then, I’m scrolling for hours through 1000s of irrelevant options."
Avg. 2 hours spent per session, 68% sessions end without purchase.
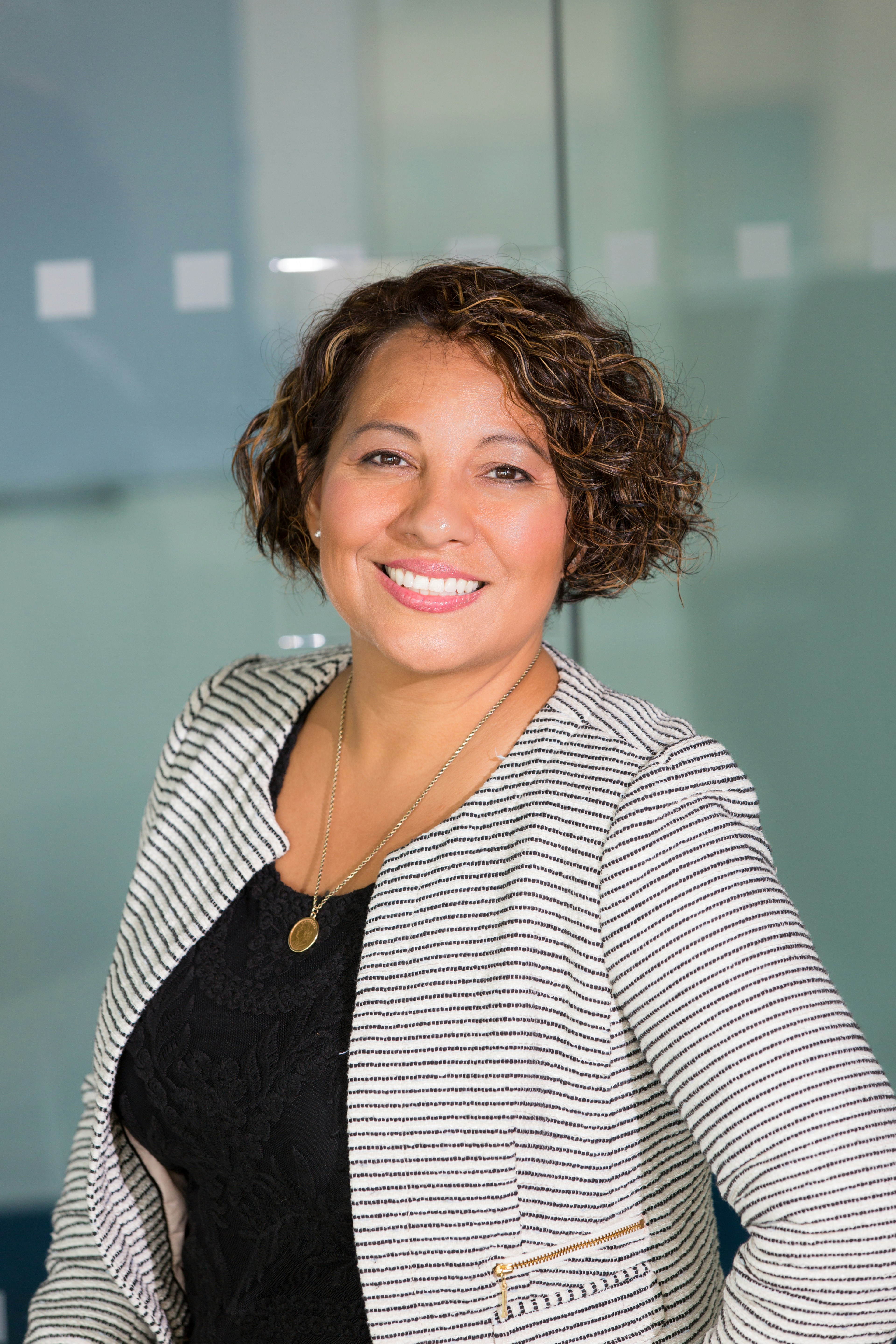
Jenny (32)
Business Owner
"My wishlist is full, but I can’t decide how to make sure it's versatile. I’m stuck in analysis paralysis—scrolling, comparing, second-guessing."
80% users abandon saved items because of decision fatigue.
JOURNEY STAGES:

USER PAINS:
"I save tons of inspiration posts, but can’t find anything similar in my budget on shopping apps."

Anita (19, Student)
"Even after applying filters, I scroll endlessly through irrelevant options."

Alexis (26, Professional)
"I keep second-guessing my wishlist—I’m stuck in analysis paralysis."
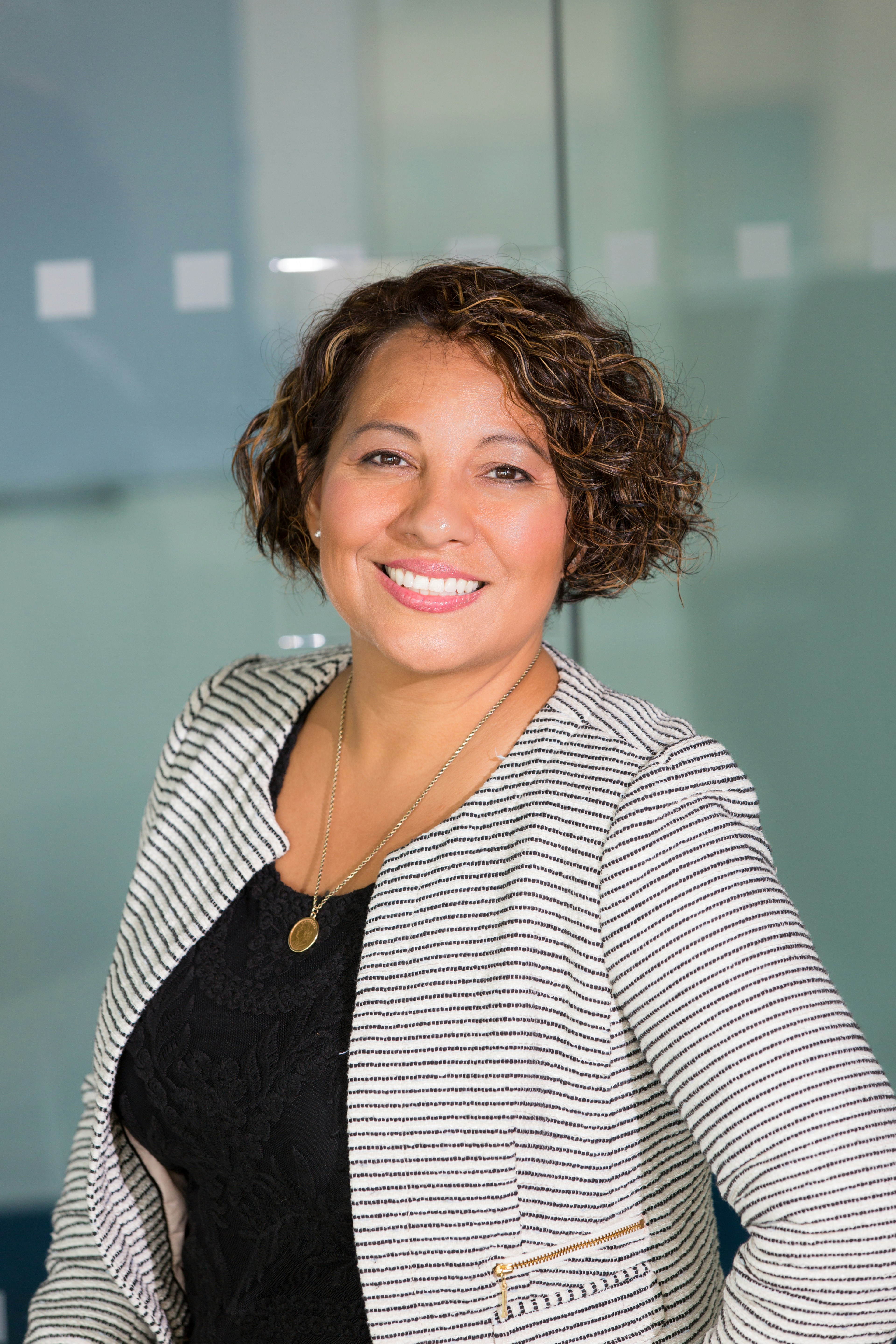
Jenny (32, Business Owner)
Key Insight: Shoppers wanted personalization and efficiency—not more options.
Here’s how I brought focus to a scattered vision
Before we could build user trust, we had to bring clarity to the product.
I started by translating the founders’ vision into a low-fidelity flow—featuring outfit planners, community boards, and social voting. While ambitious, the experience was overloaded. Once visualized, it became clear: we weren’t building a helpful assistant; we were overwhelming users with complexity.
This quick mockup became a turning point. It helped the team see that starting with everything meant delivering nothing well.


Fig. Visualizing Founder's Vision into a Low-Fid Mockup


[Next Project]
Designing a fraud detection dashboard to boost manager oversight and analyst productivity
Data Visualisation
Gamification
Interaction Design
View Full Case Study